What role does data mining play in business intelligence?
By
Last Updated May 05, 2023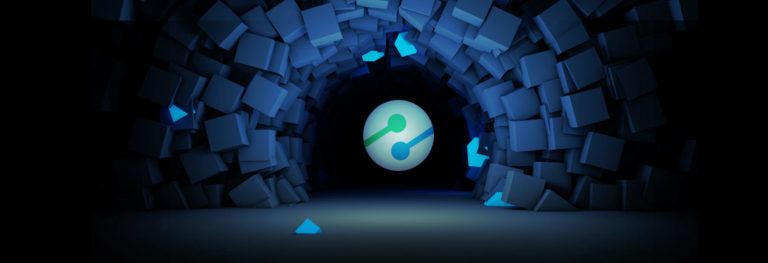
Data is the proverbial lifeblood that animates the corporate economy of the twenty-first century. And, while the mere mention of data may conjure up fanciful scenarios, the truth is that data is critical to unlocking human productivity in all aspects of life. Climate change, business failures, epidemics, and crop production can all be explained using the right data insights. For us, the availability of data shortens the learning tangent in problem-solving.
Data mining for business intelligence is important for a future-ready, self-sustaining venture, just as finding the right product-market fit is important for enterprises. It aids in future road mapping, product development, and a plethora of business processes that keep the profit wheel turning. As a result, in this article, we'll discuss articulating data mining and business intelligence topics, the importance of data mining, and how it is carried out to ensure continuous revenue flows
Table of content
Let us see What role does data mining play in business intelligence :
1. What is Business Data Mining?
2. What Role Does Data Mining Play in Business Intelligence?
3. An Introduction to Data Mining Techniques
What is Business Data Mining?
Data mining is important in business because it is used to transform raw data into meaningful, consumable, and actionable insights. Data engineers use software to look up patterns that help them analyze customers. Data sets are compared to uncover relevant metrics affecting revenue lines in order to follow up with strategies, sales improvement measures, and marketing campaign optimization.
Data mining is frequently confused and used interchangeably with data analysis and business intelligence due to the overlapping nature of the subject between data operations. However, each term is distinct from the others.
Data mining is the process of extracting information from large data sets, whereas data analysis is the process of discovering patterns in the extracted data. Data analysis includes stages such as data inspection, cleaning, transformation, and modelling. The goal is to gather information, draw conclusions, and act on it. Moving on, consider the distinctions between data mining and business intelligence.
Feature Data Mining BI Goal
To solve business problems, extract data.
Data visualization and presentation to stakeholders
Volume
Focus on smaller data sets to gain more focused insights.
Work on relational databases to gain insights at the organizational level.
Results
-
Data sets that are unique and in a usable format
-
Dashboards, pie charts, graphs, histograms, and other visual representations
-
Focus
-
Emphasize key performance indicators
-
Indicate KPI progress.
-
Tools
-
Data mining techniques are employed.
-
Data mining and data analysis are two processes that converge to help organizations generate usable and demonstrable information about their products and services.
What Role Does Data Mining Play in Business Intelligence?
The way we use data mining for business analytics and intelligence varies depending on the industry. However, there is a structure to business process management that is nearly impenetrable. Take a look at it.
Understanding of Business
If you want to be successful with data mining for business analytics, you must first identify the purpose of data mining. The following steps in the plan could address how to use the newly discovered data bits. Creating your own data mining algorithm would be a difficult task. Data comprehension
After learning about the purpose of data mining, it's time to get a feel for your data. There may be as many methods for storing and monetizing data as there are businesses. It is up to your enterprise IT strategy and practices how you create, curate, categorize, and commercialize your data.
Preparation of Data
Company data requires expert handling as one of the most important stages in the process of nurturing data mining for business intelligence. Data engineers transform data into a readable format that non-IT professionals can understand, as well as cleanse and model it based on specific attributes.
Modelling Data
To decipher hidden patterns in data, statistical algorithms are used. The finding takes a lot of trial and error.
Data Analysis
Inconsistencies in data modeling steps should be evaluated microscopically. Keep in mind that all roads (must) lead to streamlining operations and increasing profits.
Implementation
The final step is to put the findings into action in a visible way. Field trials of the recommendations should begin on a smaller scale and then be expanded to branch locations once validated.
You now understand how the accumulation of milestones leads to ground reality. Let's take a look at some of the technical aspects of data mining for business intelligence.
An Introduction to Data Mining Techniques
In this section, we'll go over each rung of the data mining ladder and how they can be used as stepping stones for future advancement.
Classification :
This is a complicated procedure that makes use of data attributes. As an example, using supermarket data to group information into categories such as groceries, dairy products, and so on is an example of data mining in business. This data can be tagged and studied to help users understand customer preferences for each line item.
Clustering :
Although it may appear to be similar to the previous step, there are some differences. Cluster groups do not have the same structure as Classification groups. Instead of specific groceries, and dairy products in the previous case, an example could be edible items, non-edible items, perishable products, and so on.
Association Regulations :
We use link variables to track patterns in this case. Continuing with our supermarket example, this could imply that customers who buy a grocery item (edible) are more likely to buy fruits (perishable). After validating Store owners can organize their shelves based on customer preferences.
Analysis of Regression :
Regression assists miners in determining the relationship between various variables in a set. It is used to forecast the likelihood of a future event. In the case of a supermarket, price points can be set based on seasonal demand, competition, and supply chain issues.
Detection of Anomalies :
Identifying outliers is the final data mining technique. There will always be anomalies in the data that must be addressed. For example, the majority of supermarket buyers are female, but for a week in (say) January, they are displaced by men. Why? Such outliers must be investigated in order to achieve a balanced approach.
The aforementioned techniques demonstrate The techniques described above demonstrate how data mining is used in business operations. Bringing this piece to a close, we can conclude that data mining and business intelligence complement each other.
Discover Enterprise BI as Never Before :
Syoft is a leading business intelligence service provider that provides unparalleled end-to-end BI services. With nearly a decade of industry experience, we've successfully launched numerous projects utilizing business intelligence techniques, with a client satisfaction rating of more than 70%. Among the services we provide in this field are:
-
Business intelligence consulting
-
Enterprise Business Intelligence Solutions
-
BI implementation, support, and upkeep
Our work history includes successful collaborations with a number of global brands.
For one of the leading telecommunications companies We deployed an effective data mining and BI solution in the United States, resulting in results such as 100% processing of customer data and an 85 percent increase in data quality and accessibility. Don't worry, if you call and ask how we can help you improve your game, we won't bite. We look forward to hearing from you (if you find our pitch interesting)!
Recent Posts
Get a Free Quote.
Lets Build Your App!
Connect
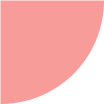
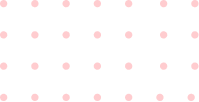